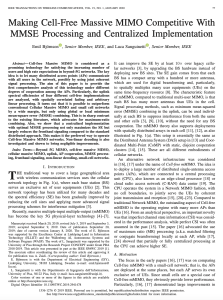
The paper “Making Cell-Free Massive MIMO Competitive with MMSE Processing and Centralized Implementation” that I’ve authored together with Luca Sanguinetti has been awarded the 2022 IEEE Marconi Prize Paper Award in Wireless Communications. This is a great honor that places the paper on the same list as many seminal papers published in the IEEE Transactions on Wireless Communications.
I will take this opportunity to elaborate on five things that I learned while writing the paper. The basic premise is that we analyze the uplink of a system with many distributed access points (APs) that serve a collection of user devices at the same time and frequency. We compared the data rates that can be achieved depending on how deeply the APs are collaborating, from Level 1 (cellular network with no cooperation) to Level 4 (cell-free network with centralized computations based on complete signal knowledge). We also compared maximum ratio (MR) processing of the received signals with local and centralized forms of minimum mean-squared error (MMSE) processing.
I learned the following five things:
- MMSE processing always outperforms MR processing. This might seem obvious, since the former scheme can suppress interference, but the really surprising thing was that the performance difference is large even for single-antenna APs that operate distributively. The reason is that MMSE processing provides much more channel hardening.
- Distributed MR processing is the worst-case scenario. Many of the early works on cell-free massive MIMO assumed distributed MR processing and focused on developing advanced power control algorithms. We demonstrated that one can achieve better performance with MMSE processing and rudimentary power control; thus, when designing a cell-free system, the choice of processing scheme is of primary importance, while the choice of power control is secondary.
- Linear uplink processing is nearly optimal. In a fully centralized implementation, it is possible to implement non-linear processing schemes for signal detection; in particular, successive interference cancellation could be used. We showed that this approach only increases the sum rate by a few percent, which isn’t enough to motivate the increased complexity. The reason is that we seldom have any strong interfering signals, just many weakly interfering signals.
- Distributed processing increases fronthaul signaling. Since the received signals are distributed over the APs, it might seem logical that one can reduce the fronthaul signaling by also doing parts of the processing distributively. This is not the case in the intended operating regime of cell-free massive MIMO, where each AP serves more or equally many users than it has antennas. In this case, fewer parameters need to be sent over the fronthaul when making a centralized implementation!
- Max-min fairness is a terrible performance goal. While a key motivation behind cell-free massive MIMO is to even out the performance variations in the system, compared to cellular networks, we shouldn’t strive for exact uniformity. To put it differently, the user with the worst channel conditions in the country shouldn’t dictate the performance of everyone else! Several early works on the topic focused on max-min fairness optimization and showed very promising simulation results, but when I attempted to reproduce these results, I noticed that they were obtained by terminating the optimization algorithms long before the max-min fairness solution was found. This indicates that we need a performance goal based on relative fairness (proportional fairness?) instead of the overly strict max-min fairness goal.
Since the paper was written in 2019, I have treated centralized MMSE processing as the golden standard for cell-free massive MIMO. I have continued looking for ways to reduce the fronthaul signaling while making use of distributed computational resources (that likely will be available in practice). I will mention two recent papers in this direction. The first is “MMSE-Optimal Sequential Processing for Cell-Free Massive MIMO With Radio Stripes“, which shows that one can implement centralized MMSE processing in a distributed/sequential manner, if the fronthaul is sequential. The paper “Team MMSE Precoding with Applications to Cell-free Massive MIMO” develops a methodology for dealing with the corresponding downlink problem, which is more challenging due to power and causality constraints.
Finally, let me thank IEEE ComSoc for not only giving us the Marconi Prize Paper Award but also producing the following nice video about the paper:
Thanks for sharing
For your first point in the comment
1- how can the AP do MMSE processing with one antenna? I mean is that feasible? The model is y=hx+d+n to get x from y where n is noise and d is interference?
2- it is mentioned in the blog that MMSE is able to achieve better hardening than MR but as far as I know hardening is a property of the channel not the processing steps so how is that?
3- how can you prove mathematicaly that hardening from MMSE is better than that of MR (assuming hardening is related to the receive processing )
4- what about the cost of operations I mean this can’t be simulated the cost of deploying tens of cell free AP equipped with multiple antennas to provide certain QoS which is better from this pov us it cell or cell free
5- what about the planning isn’t it like femto cells? Interference is easily achieved here with no way of proper planning since there is no boundaries because there are no cells.
Thanks
Hi Yasser,
You can find detailed answers to these questions in the paper and in my book on cell-free massive MIMO, but let me give you some brief answers.
1. One just has the apply the MMSE combining formula, which is a scalar in this case.
2. Hardening is the property that the effective channel after precoding/combining is nearly deterministic. The amount of channel hardening depends on the channel properties and the choice of precoding/combining. Many papers and studying channel hardening for the special case of MR processing and perfect CSI, but it is a more general property.
3. MR and MMSE combining will be equal up to a scaling factor in the case with a single antenna, but that scaling factor is random and makes a big difference. Figure 6.7(a) in https://arxiv.org/pdf/2108.02541.pdf illustrates the difference in the distribution of the signal after combining (actually precoding in this example, but it is the same thing).
4. You can find an analysis of the number of operations in Chapter 5 and 6 of the book https://arxiv.org/pdf/2108.02541.pdf
Local MMSE combining has almost the same complexity as MR combining, since each AP has only a few antennas. Centralized MMSE combining is more complex.
5. A dense deployment of femtocells will lead to much interference between the cells, which cell-free massive MIMO is resolving. This is further discussed in Section 1 and Section 5.4.3 of the book.
Nicely explained, thanks Emil. I wish every paper came with a nice explanatory text and video!
Emil, congratulations! nicely done!
A key feature for Cell-Free Massive MIMO is having enough APs to ensure 100% coverage, i.e., none of the intended users are left “in the country”. For the set of intended users, how is max-min fairness “a terrible performance goal”?
Thank you!
What I mean is the following: Coverage is defined as having an SNR above a minimum threshold. In a large network, a few users will have an SNR close to the threshold, while many users will have much larger SNRs. The latter ones are sensitive to interference, while the former ones are not.
If we strictly enforce max-min fairness, the users with low SNRs will barely see a performance improvement (they weren’t interference-limited to start with), while all the users with much higher SNRs will be forced to get a performance that matches the users with low SNR. This happens even if their influence on the weakest users is almost zero (but not exactly zero).
Under these conditions, we will not get “uniformly great service for everyone” but uniformly bad service for everyone…
Here is an interesting observation: The two thick curves in Figure 4(b) are supposed to match with Figure 6 in “Cell-free Massive MIMO versus small cells”, but your paper actually shows much better results. The reason is that your max-min fairness optimization algorithm terminates before it reaches the point where everyone gets exactly the same rate. This shows that by sacrificing a tiny amount of the worst user’s performance, one can improve tremendously for many other users. This is another reason why I think max-min fairness is a terrible performance goal: it is too strict.
Just wanted to congratulate both Prof. Björnson and Prof. Sanguinetti for the Marconi Prize Paper Award. This paper has served as a core reference for my own research, and it’s been truly insightful. Thank you both for your contributions!
Hi Prof. Björnson,
Congratulations and thanks for your sharing.
Could I ask one question that I do not understand well in the paper?
As you mentioned in Level 3 where you used the channel statistics, the vector g_ki is the weighted channel which is v times h. The effective channel is unknown at the CPU, but why we can get its average? In my view the expectation of the g_kk includes the real channel h, so how the AP knows the real channel coefficients?
In other words, we can know the vector v based on the estimate channel, and the received signals at the AP. But I don not know how to calculate the mean value of g_kk.
Information-theoretic studies always assume that the statistics are known, because the capacity is achieved by transmitting infinitely long codes. Hence, there will be plenty of time for estimating a few statistical parameters by gathering realizations over many coherence blocks.
In practice, where the codes are of finite length, it might be more challenging to do this. So you are right in questioning that. One positive thing is that we might not need to obtain every statistical coefficient in practice, because many only appear in summation expressions.
Thanks so much.
Sir I asked earlier also can I combine cell-free massive MIMO with NOMA to achieve spectral efficiency and energy efficiency. Please answer this in elaborative way
Cell-free massive MIMO systems are meant to have many more antennas than users, which is a situation in which NOMA doesn’t provide much performance gains. A simulation example can be found in Figure 5 in https://arxiv.org/pdf/1903.10611 You can compare L4 (MMSE) with L4 (MMSE-SIC), where the latter is a NOMA scheme.
My general opinion is that NOMA is an outdated technology now when multi-user MIMO is used everywhere. It seldom gives noticeable performance gains when comparing with state-of-the-art MIMO methods and it adds a lot of complexity.
I recommend you to read Chapter 6 in my book, where I elaborate on NOMA vs. OMA and multi-user MIMO: https://www.nowpublishers.com/article/BookDetails/9781638283140